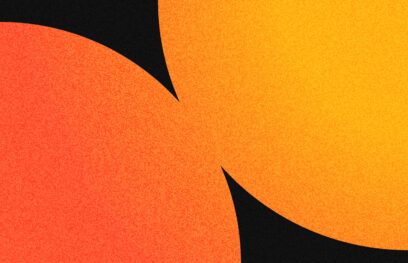
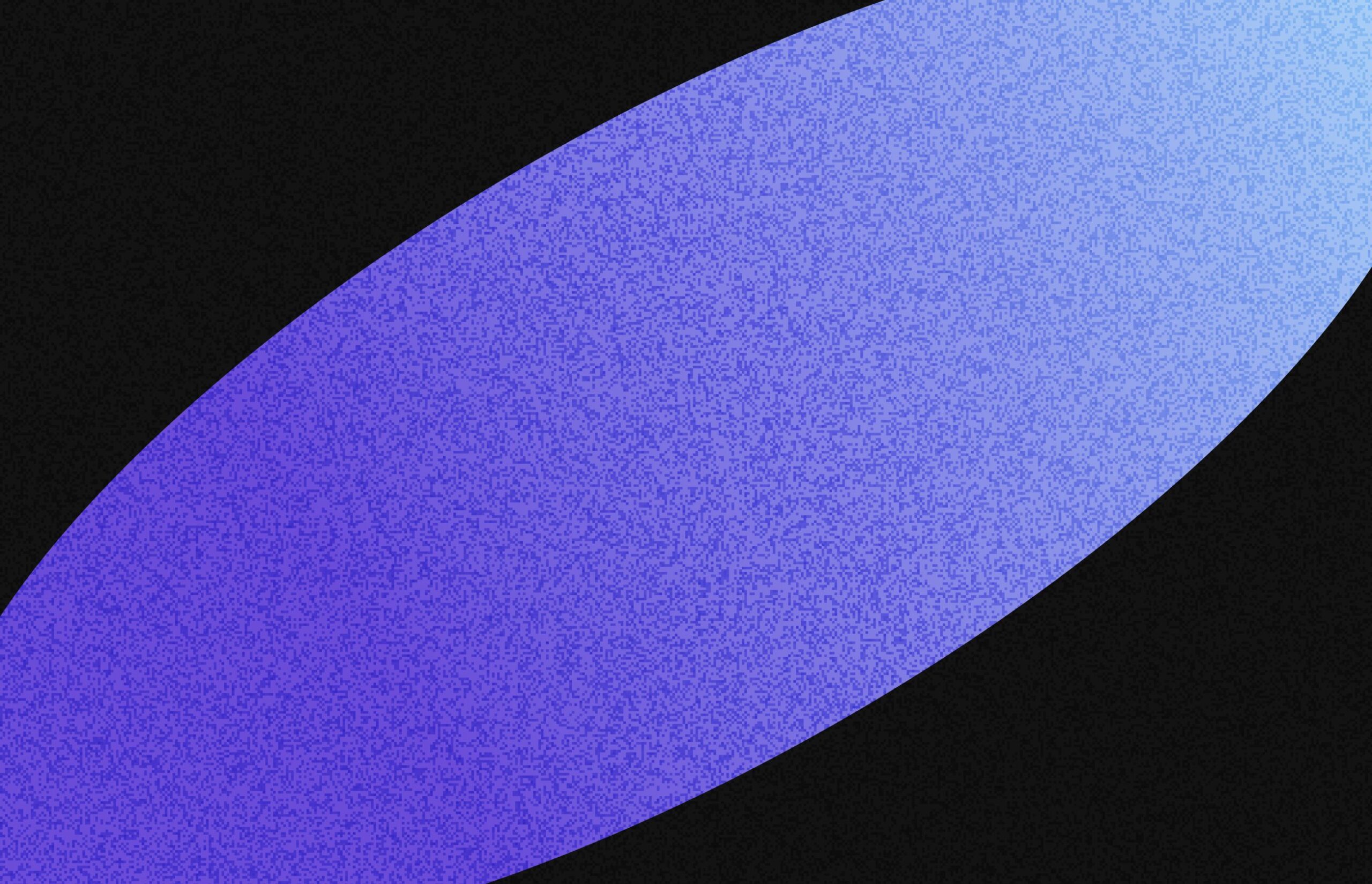
AI is evolving, fast. The question has officially transitioned from “can we” to “should we” as tech companies face pressure to incorporate AI into their products. What was once seen as optional is becoming mandatory for many, calling for the need to understand and embrace AI in product design. In this article, we’ll share our framework to make user-centered design decisions, enabling you to develop better AI-powered products.
The Shift to User-Centered Design
With a new technology like AI, the endless possibilities are exciting—to the point of becoming distractions. Remember all the useless apps we developed when mobile app technology was new, like the beer-drinking simulator?
The real innovation comes when we consider the needs of users and apply the new opportunities of the technology, thoughtfully and intentionally. Today’s products that showcase AI’s excellence have something in common: outstanding user experience. But how do you get an outstanding user experience with AI?
The AI Design Strategy Framework
It’s not just about how cool the technology is behind the product, but how it serves the needs of your users. When making design decisions, always start with the three Cs of AI in product design.
Capabilities & Constraints
Despite continuous innovation, it’s still important to balance the opportunities and limitations of AI to make informed design choices.
Ask yourself:
- What AI functionality is possible today?
- What isn’t feasible to design right now, but could go on the roadmap for later?
Clarity
Communication is key, so explain how AI helps users’ processes. If users don’t understand how AI helps, they won’t its value in your product. You’ll also need to address the gaps to fill, as the development of AI continues.
Ask yourself:
- What does AI accomplish for the user?
- Are the use cases obvious to the user?
- What can AI not do that we can solve for?
Along with the three Cs, consider the level of magic and user input.
Yes, magic. AI has a wow factor that attracts and pleases users—if you choose. AI that works in the background and is invisible to users has a low magic level, while AI at the forefront of the user experience has a higher level. And keep in mind, having AI in the background isn’t a bad thing.
Ask yourself:
- Does it make sense to wow users with AI in your product, or have it work in the background?
User input is the amount of effort required of the user to utilize AI in your product. This is why clarity is important—a product with high user input without clear instruction renders AI unusable.
Ask yourself:
- How much effort is required of the users to experience the benefit of AI?
Evaluating AI Design Enhancements
Now that you have the framework, let’s take a look at some of AI’s most common design enhancements.
Generation
Generative AI is what has been hitting the news over the last year: Give the AI engine a prompt, and it will create what you’ve instructed in formats ranging from text and images to video and audio. While generative AI can create amazing assets, be aware that it does have serious issues with inaccuracy and correctness in fine details.
Consider using generation in your software when:
- Your users need help turning ideas into something tangible
- Your users have a “blank page” problem and need fast suggestions
Be careful about using generation when:
- Accuracy and correctness are important
Suggestion
Suggestion makes recommendations to the user on the next step to take based on machine learning and past data. For example, imagine an email marketing platform that suggests follow-up email formats that perform well.
Suggestion is extremely powerful: it elevates your software from just helping users perform tasks to helping them be more productive, brings awareness to features they may not know about and establishes your company as a thought leader in the domain. On the other hand, it can be subjective: good suggestions for some users may not be appropriate for others.
Consider using suggestion when:
- Your users seek assistance for being more productive
- Your features are hard to find
- You want users to see your software as “the expert”
Be careful about using suggestion:
- In situations where suggestions could lead the user to make questionable decisions
- Where suggestions are very subjective and could turn users off to your software
Curation
Curation features have been present in the market for quite some time: Netflix and Spotify use curation to create custom recommendations specific to users’ tastes. Curation uses data about user behavior and consumption habits to create personalized lists.
Consider using curation when:
- You have a large amount of content and a large amount of data on user preferences
- It’s hard for users to sift through content to find what interests them
- Your users want personalization and could engage even more with customized choices
Be careful about using curation when:
- You don’t have enough data about user preferences
- Your users want to see all the options available to them and enjoy having choices
Prediction
Prediction utilizes past user behavior data to forecast or predict shortcuts. They can seem like magic to users by being extremely personalized and saving them time. An example is Apple CarPlay showing directions to Starbucks every Wednesday when you get in your car because your past behavior has been to go to Starbucks on Wednesdays. One constraint for using prediction is that patterns change, and when predictions are inaccurate or used incorrectly, it decreases the credibility of the software.
Consider using prediction when:
- You have a large amount of data on behaviors and results
- Predictions can draw the user’s attention to a result they may not have considered
- Predictions can reduce the user’s workload
Be careful about using prediction when:
- An incorrect prediction could have a critical or catastrophic outcome
- When the user’s patterns change too often for a prediction to be accurate
Analysis & Summarization
Analysis and summarization can save users time by synthesizing large amounts of information. Amazon is using analysis and summarization by providing summaries of user reviews for products so that users don’t have to read hundreds to understand overall sentiment. Because AI has issues with inaccuracy, it’s important to be careful to use summarization in the appropriate situations.
Consider using summarization when:
- Users need to go through a lot of data to do their own explicit or implicit synthesis and summarization
- A general summary is appropriate and helpful
Be careful using summarization when:
- Relying on the accuracy of the summary is critical, personally or from a business perspective
- The high-level, subjective nature of the summary will be unhelpful or frustrating
Recognition
Recognition is where the AI identifies images or text. For example, Google Photo or Apple Photo recognizes the subject of a photo is a dog, and allows you to search for all images that contain a dog. These features always feel like magic to the user because they involve the human-like capacity to recognize animals, objects, people and text. The main constraint for AI recognition is accuracy. Recognition has some truly exciting use cases in health and personal safety, but it is important that the product frames the recognition the right way and provides the right experience to handle ambiguity and uncertainty.
Consider using recognition when:
- You have content that users need to transform to other modalities, like from image to text
- A little inaccuracy is okay in the recognition
Be careful using recognition when:
- The stakes of the recognition are high, as in health or personal safety situations
- Training data is incomplete or inaccurate
Task Execution
Task execution is when AI can handle small jobs for the user. This use of AI can be tailored to the software and the needs of users. One example of this is in Miro, an online whiteboard and workshop software, that automatically themes a set of post-it notes. One challenge is ensuring that users are aware that such features are available to them. Don’t forget your “feature onboarding” to help users understand that these features exist. Another challenge is using AI task execution for high-stakes tasks. If you do automate high stakes tasks, ensure that you are giving the human the chance to check the AI’s work.
Consider using task execution when:
- You have tedious, boring or time-consuming tasks that you can automate through AI
- You can help users get to the more strategic parts of their work in the software quickly by automating upfront tasks
Be careful using task execution when:
- The stakes of the task are high
- Users enjoy doing the tasks
Summary: Considerations for AI in Product Design
There’s much to consider, but now you have a place to start.
Put users first.
- Will AI in your product add the right value to your users?
- Is it designed to meet their expectations?
Understand capabilities and constraints.
- How do you design within the practical boundaries of AI?
- How can you prioritize what to design now vs. add to the roadmap?
Design for clarity.
- What does AI do for the user?
- How does AI help users achieve their goals?
Know when to turn up the magic.
- How much are you “wowing” users with AI in design?
- When is it appropriate for AI work in the background?
Consider the level of user input required.
- How much effort is required from users to see the benefit of AI?
- To users, what is worth spending the energy on?
At Innovatemap, we’ve helped multiple companies design with AI in the unique context of their products. For something that may be brand new to your team, we’re a partner who’s worked through this before.
Learn more about how Innovatemap can help you enhance your product with AI.